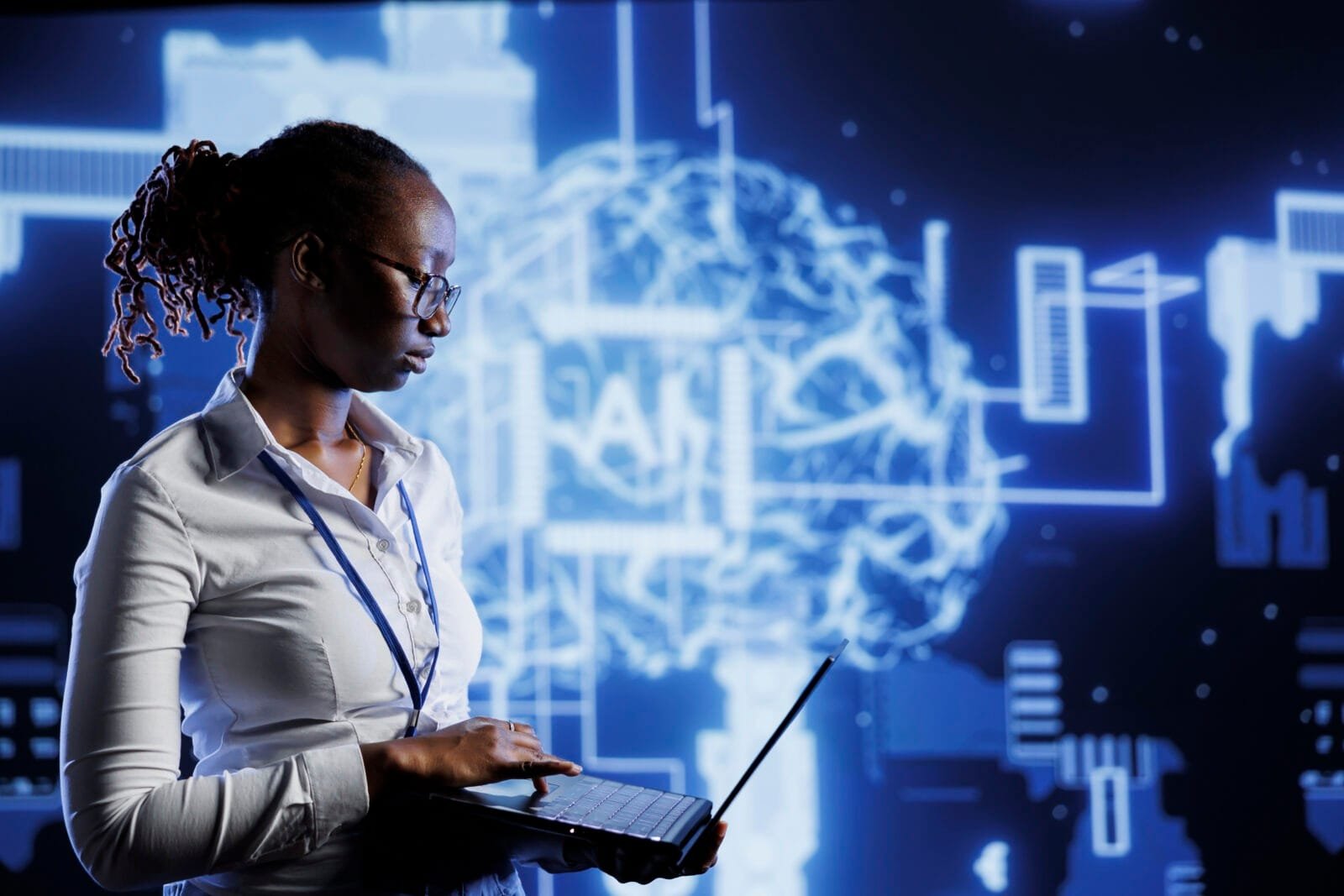
The advent of artificial intelligence (AI) has sparked revolutionary changes across various sectors, but no other aspect of AI seems as promising and transformative as generative AI (gen AI). For the technology, media, and telecommunications (TMT) industries, gen AI stands as a powerful tool poised to redefine operational models, drive growth, and enhance customer experiences. Yet, while its potential is immense, the path to unlocking this potential is fraught with challenges and uncertainty.
Generative AI’s rise presents a monumental opportunity, as highlighted by McKinsey’s research. It estimates that gen AI could add between $2.6 trillion and $4.4 trillion to the global economy annually, while increasing the total impact of AI by 15 to 40 percent. For the TMT sector, the stakes are particularly high, with new gen AI applications expected to create an impact ranging from $380 billion to $690 billion. This breaks down into $60 billion to $100 billion in telecommunications, $80 billion to $130 billion in media, and a staggering $240 billion to $460 billion in high tech.
The pace at which gen AI is evolving means that in the next few years, technologies and processes not integrated with AI may become obsolete. Companies are now at a critical juncture: they must either adapt to this wave of AI-driven transformation or risk falling behind. For forward-looking executives, understanding the full potential of gen AI and how to incorporate it into their operations will be essential to maintaining competitiveness and relevance in this rapidly shifting landscape.
Gen AI’s Use Cases in TMT
McKinsey’s research has uncovered more than 100 gen AI use cases across seven business domains within the TMT sector. These cases span customer care, sales, network operations, IT, support functions, and more. The most significant impacts are expected in customer care and sales, which together could account for approximately 70 percent of the total impact in telecommunications.
In customer care, gen AI holds the potential to automate up to 50 percent of interactions, leading to productivity improvements of 30 to 45 percent while enhancing customer satisfaction scores. Personalized product recommendations, tailored marketing campaigns, and improved sales effectiveness could lead to revenue increases of 3 to 5 percent. Meanwhile, on the labor side, up to 70 percent of repetitive tasks could be automated, driving improvements in both efficiency and employee satisfaction.
Gen AI’s impact extends beyond customer-facing functions. Knowledge search, validation, and synthesis, where 60 percent of activities could be automated, offer potential for new efficiencies. Furthermore, gen AI can enhance the productivity of developers, with productivity gains ranging from 20 to 45 percent as coding tasks and debugging processes are streamlined.
These areas provide fertile ground for the development of use cases that can drive meaningful impact in the TMT sector. However, the challenge lies in moving from conceptual roadmaps to scaled implementations that deliver measurable results.
Becoming an AI-Native Organization at Scale
One of the key challenges that companies face is how to scale gen AI across their operations effectively. The path from a pilot stage to large-scale deployment requires a comprehensive strategy that encompasses technology, data, talent, and governance.
To become an AI-native organization, companies need to invest in high-quality data and build a robust data architecture capable of supporting gen AI models. This involves incorporating capabilities such as vector databases and advanced data pre- and post-processing pipelines. Moreover, companies need to focus on talent— recruiting and developing data science and analytics experts who can work closely with AI tools to drive business outcomes.
A successful AI-native transformation also involves developing an operating model that leverages the strengths of both humans and machines. This model should be rooted in agility, flexibility, and continuous learning, with a strong focus on collaboration between different teams across the organization. Moreover, it should be supported by a governance framework that ensures transparency, ethical AI use, and compliance with evolving regulations.
Building the Foundation for Success in TMT
As generative AI (gen AI) begins to shape the future of the TMT industry, organizations must undergo a profound transformation to fully capitalize on its potential. This transformation, known as becoming “AI-native,” is more than just adopting AI tools. It involves a fundamental shift in how businesses operate, make decisions, and interact with customers.
The backbone of any AI-native organization is its technology infrastructure. In the TMT sector, this means deploying scalable AI architectures that can process large volumes of data in real-time. Companies need to invest in advanced machine learning models, cloud computing capabilities, and AI-driven platforms that can deliver rapid insights and automate decision-making processes. Building a robust infrastructure also requires integrating gen AI into existing systems and ensuring compatibility with legacy technologies.
For instance, in telecommunications, AI-powered tools can optimize network operations, automate customer service interactions, and improve call routing efficiency. In media, AI can streamline content creation and distribution, personalizing user experiences based on individual preferences. By embedding gen AI into their technology stack, TMT companies can drive innovation and operational efficiency at scale.
Data is the fuel that powers AI. For generative AI to deliver meaningful results, companies need to have high-quality, structured, and unstructured data at their disposal. This means investing in data collection, storage, and management systems that ensure accuracy, completeness, and real-time accessibility. Data pipelines, vector databases, and advanced pre- and post-processing capabilities play a crucial role in refining the datasets used to train AI models.
Overcoming the Challenges of Gen AI Implementation
As promising as gen AI is, implementing it at scale comes with a unique set of challenges. Organizational readiness is a critical factor— leaders need to foster a culture that embraces innovation, supports experimentation, and is open to change. A major obstacle is the shortage of AI and data science talent. Additionally, C-level executives often hesitate to prioritize AI initiatives, either due to a lack of understanding or uncertainty about return on investment (ROI).
Another challenge lies in data. Many companies struggle to find the large, high-quality datasets needed to train AI models effectively. Data privacy and security concerns, especially in highly regulated industries like telecommunications, further complicate the situation. Moreover, the interpretability of AI models is another concern. Ensuring that AI-generated outputs are reliable and understandable to end-users is critical for building trust in the technology.
To address these challenges, companies must focus on building a system of protocols and guardrails. For example, implementing “moderation” models can help check AI outputs for risks such as bias or inaccuracies, ensuring that customers and employees receive consistent and reliable responses. At the same time, change management is key. Companies need to involve end-users early in the model development process and deeply embed the technology into their workflows to drive adoption and maximize impact.
Navigating the Regulatory Hurdles
The regulatory environment for AI and gen AI is evolving rapidly. As governments and regulatory bodies work to establish rules and frameworks for AI, companies must stay ahead of these developments to ensure compliance and mitigate risks.
One of the key areas of concern is data privacy. With AI models relying on vast amounts of personal data, companies need to ensure that their data collection and usage practices align with privacy regulations such as the General Data Protection Regulation (GDPR) in Europe and the California Consumer Privacy Act (CCPA) in the United States. Additionally, there is growing scrutiny around the ethical use of AI, particularly when it comes to issues such as algorithmic bias, transparency, and accountability.
To navigate this complex regulatory landscape, companies should establish a dedicated governance team responsible for monitoring regulatory developments, conducting risk assessments, and ensuring compliance with AI-related regulations. This team should work closely with legal, compliance, and data teams to develop policies and procedures that safeguard customer data and ensure ethical AI practices.
The Future of Gen AI in TMT
The future of gen AI in the TMT sector is bright, with new capabilities and advancements constantly emerging. One of the most exciting developments is the ability of gen AI to analyze and comprehend images and audio. This opens up new possibilities for applications in fields such as media production, telecommunications, and content creation. Additionally, the ecosystem for generative pretrained transformers (GPT) continues to expand, with marketplaces for GPT models allowing companies to access pre-trained models that can be customized for specific use cases.
For TMT leaders, the stakes are high. The next few years will be critical in determining whether companies can move beyond isolated AI use cases to full-scale implementations that drive significant business impact. Those who succeed will not only capture the economic potential of gen AI but also position themselves as leaders in the digital economy.
The rise of generative AI represents a defining moment for the TMT sector. While the technology’s potential is immense, realizing this potential requires a clear vision, a decisive strategy, and a willingness to embrace innovation. Companies must invest in data, talent, and technology while building the governance structures needed to manage AI-related risks effectively. As they do so, they must also be prepared to navigate the evolving regulatory requirements, ensuring that their AI practices are ethical, transparent, and compliant with data privacy regulations. In the coming years, those TMT organizations that successfully integrate gen AI into their operations will be well-positioned to lead the industry into the future.